1. Traffic Risk Prediction involved Children Pedestrians in Reading, PA
R; JavaScript; Google Cloud Platform
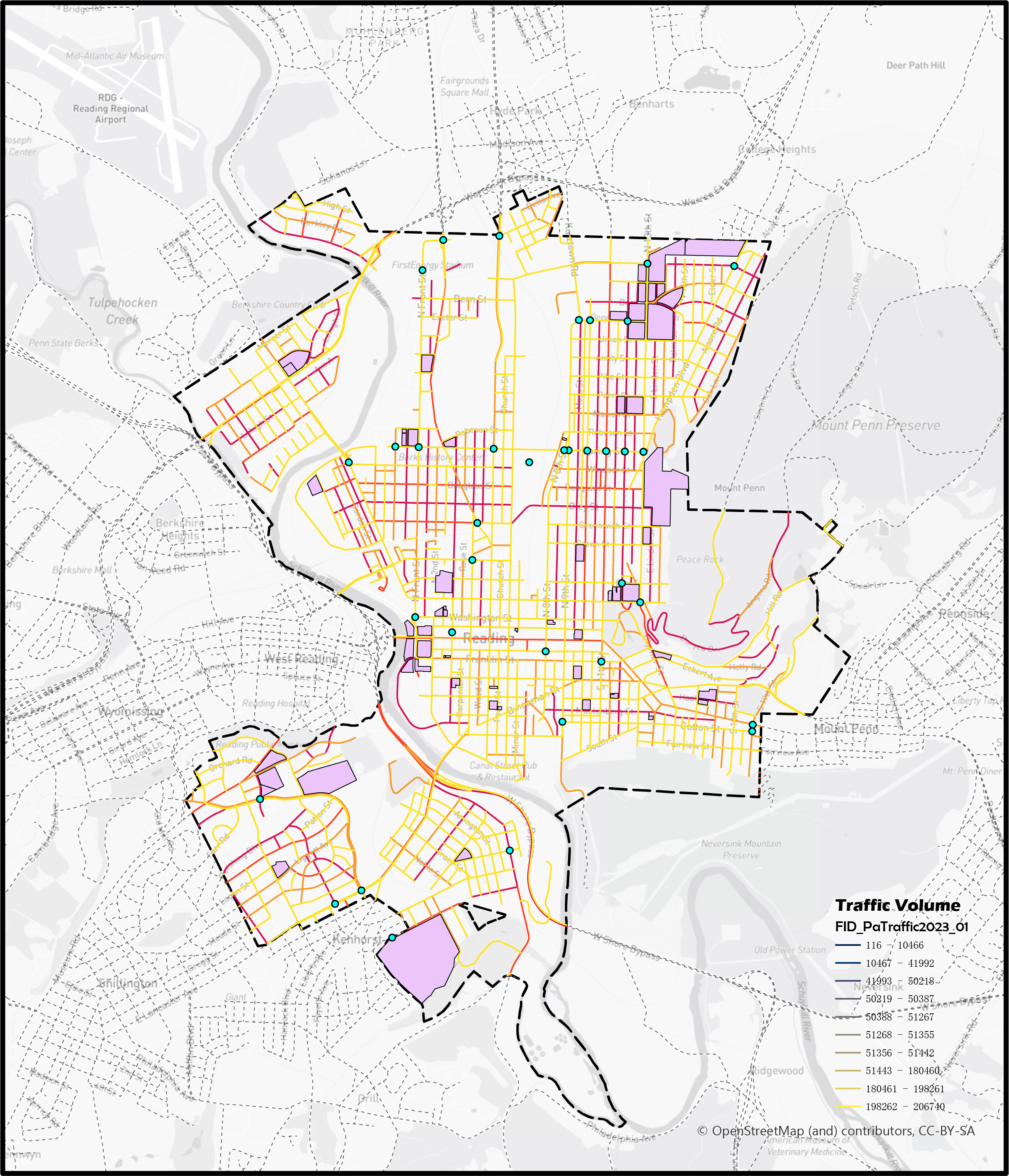
In the United States, children remains 20% of total 38,680 car crashes records in 2020, which facing a high risk of being involved in traffic accidents. This research project aims to address this issue by focusing on this vulnerable group and developing a precise model to identify potential risks and implement protective measures.
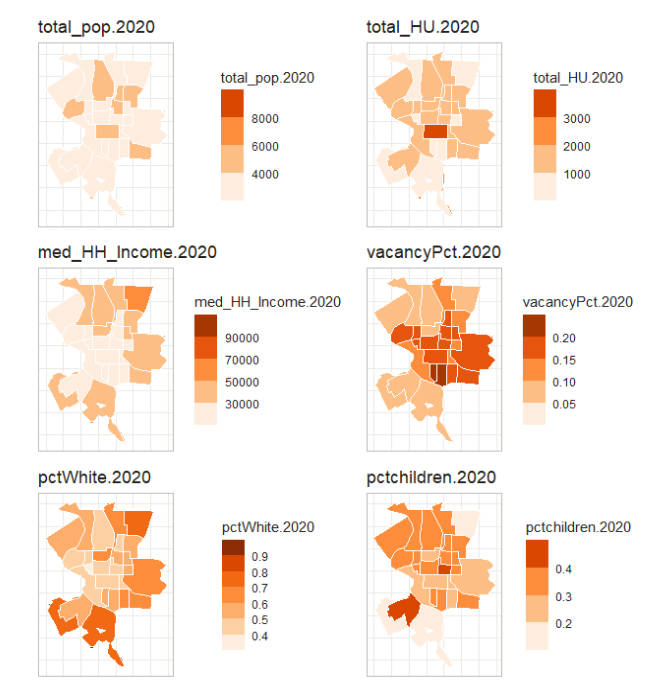
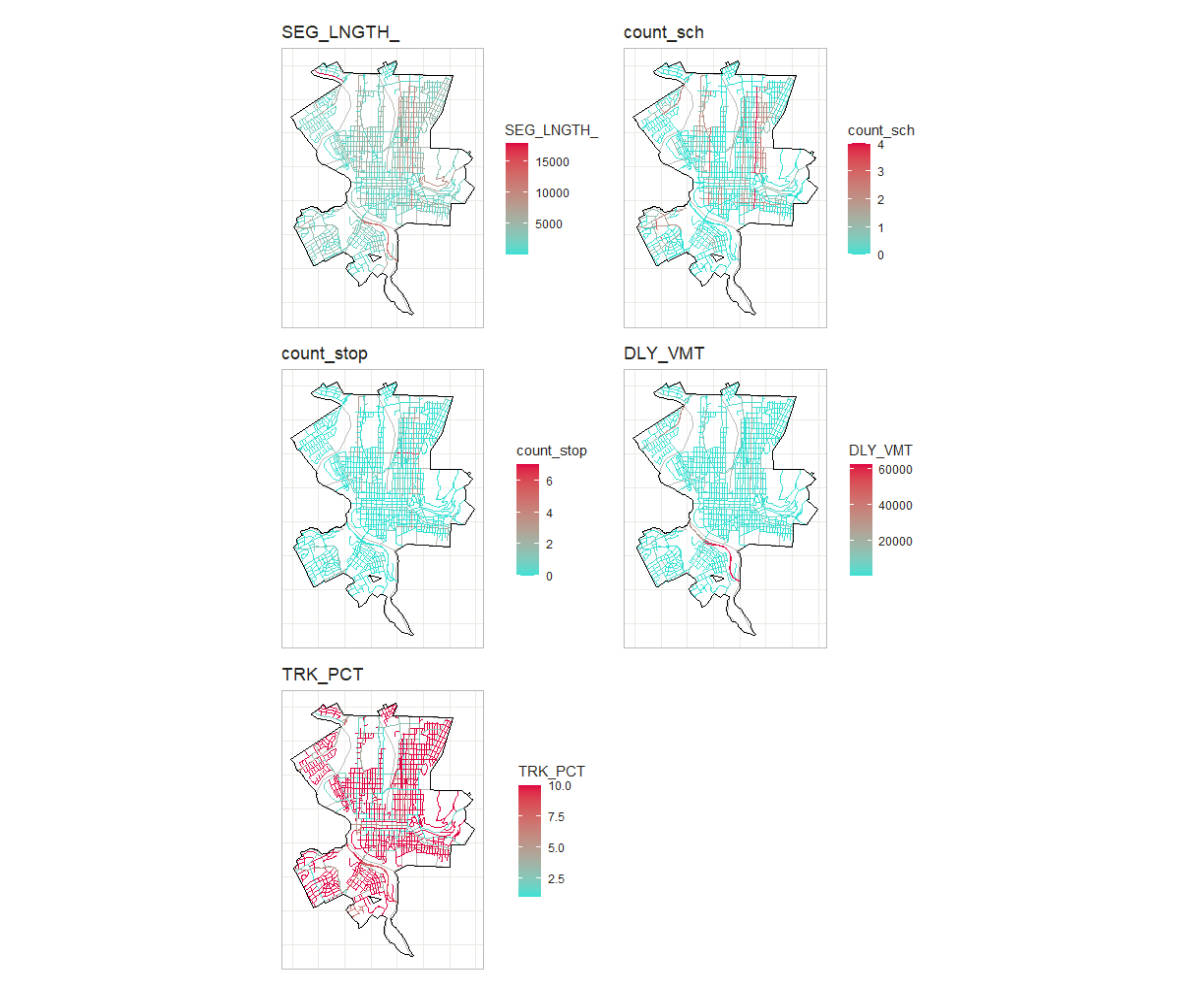
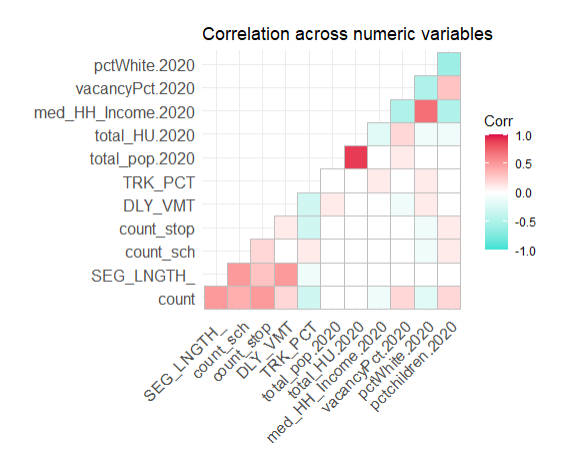
Crash Data Aggre
gated on Census Tract Level and Road Segment Level
Variables Correlation Matrix
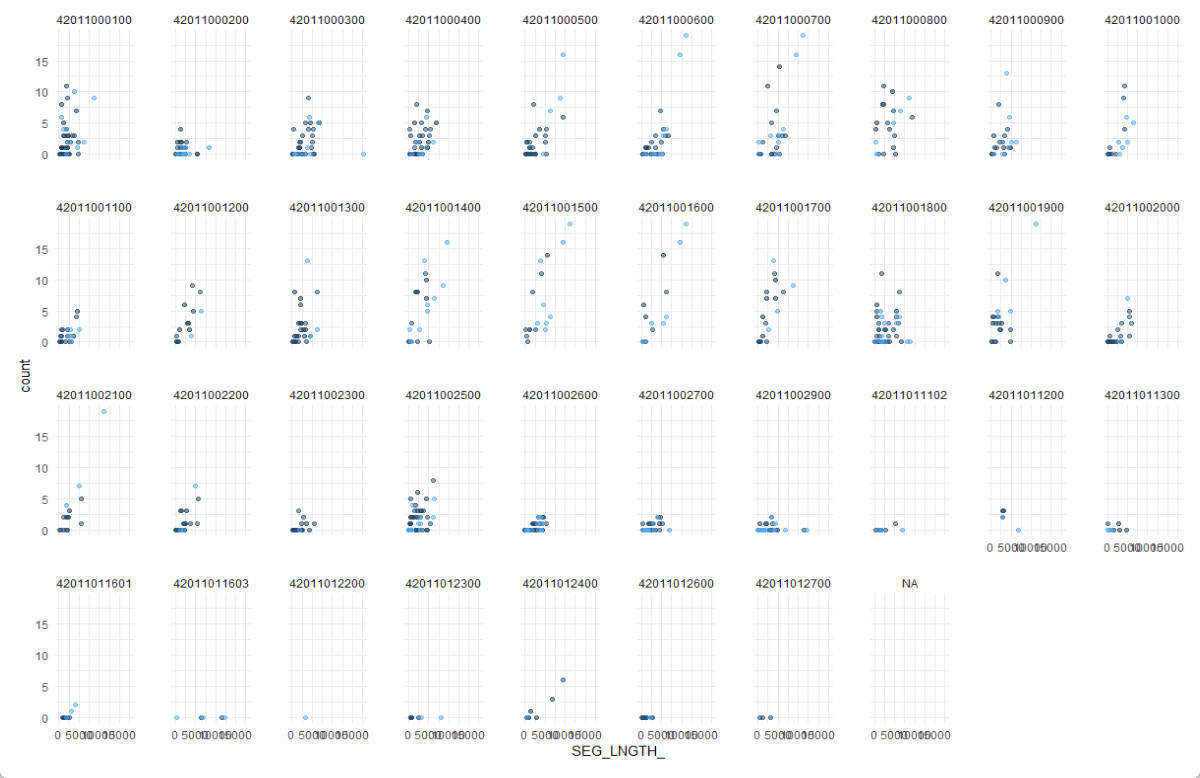
Data Explorer by Census Tract ID
Methods
Deployed a multilevel model account for hierarchical structure of individual crashes history and neighborhoods by modeling the variation in car crashes at each level, estimating the effects of predictors, and predicting the potential possibility on each road segment.
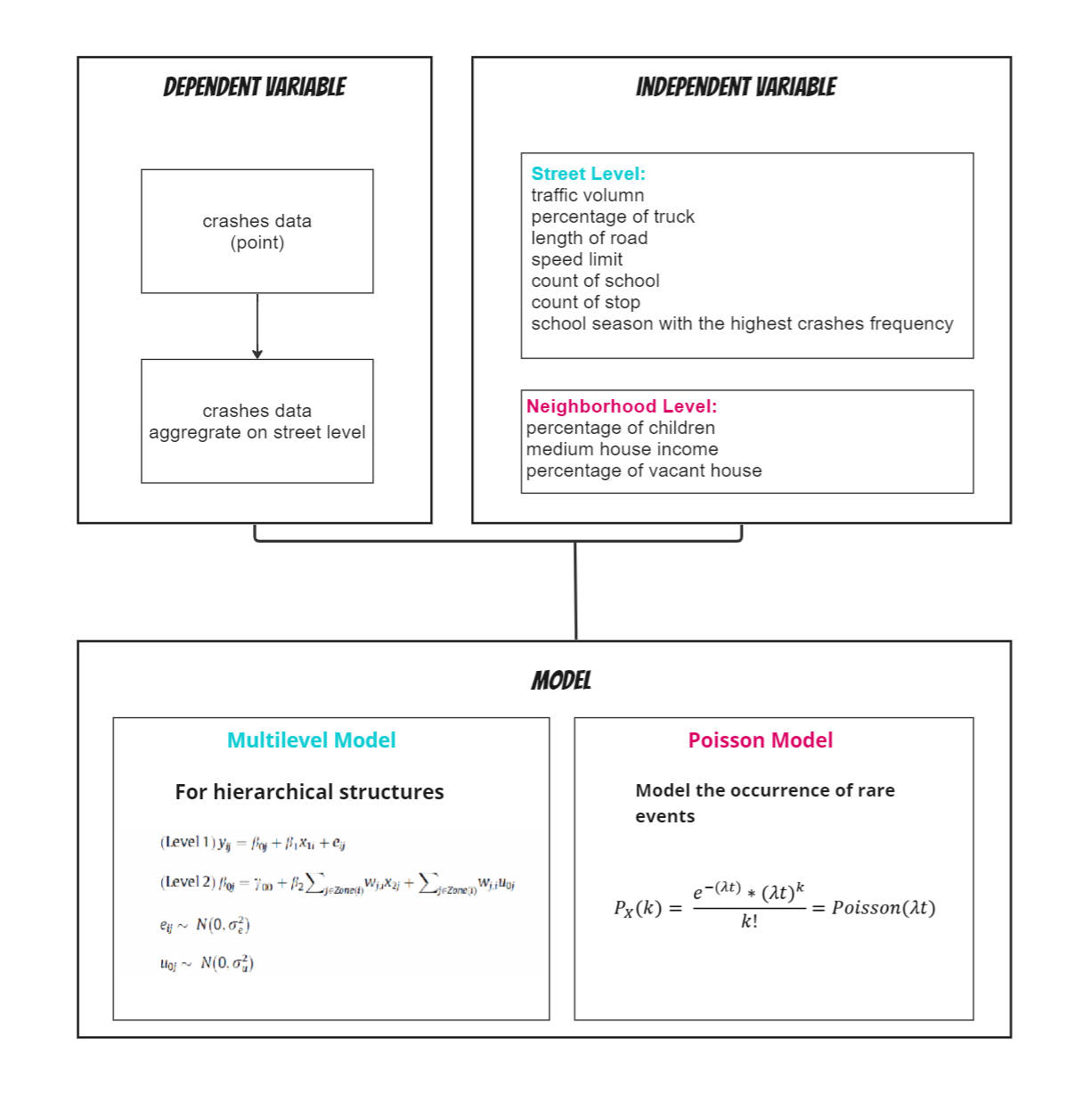
Data Pipeline
Leveraged Google Cloud Platform to ingest and process streaming data from PennDOT( Pennsylvania Department of Transportation ). By automating the data collection and analysis process, a data pipeline can provide real-time or near real-time insights into traffic patterns and risk factors. This can help transportation agencies and emergency services respond more quickly to accidents or other incidents.
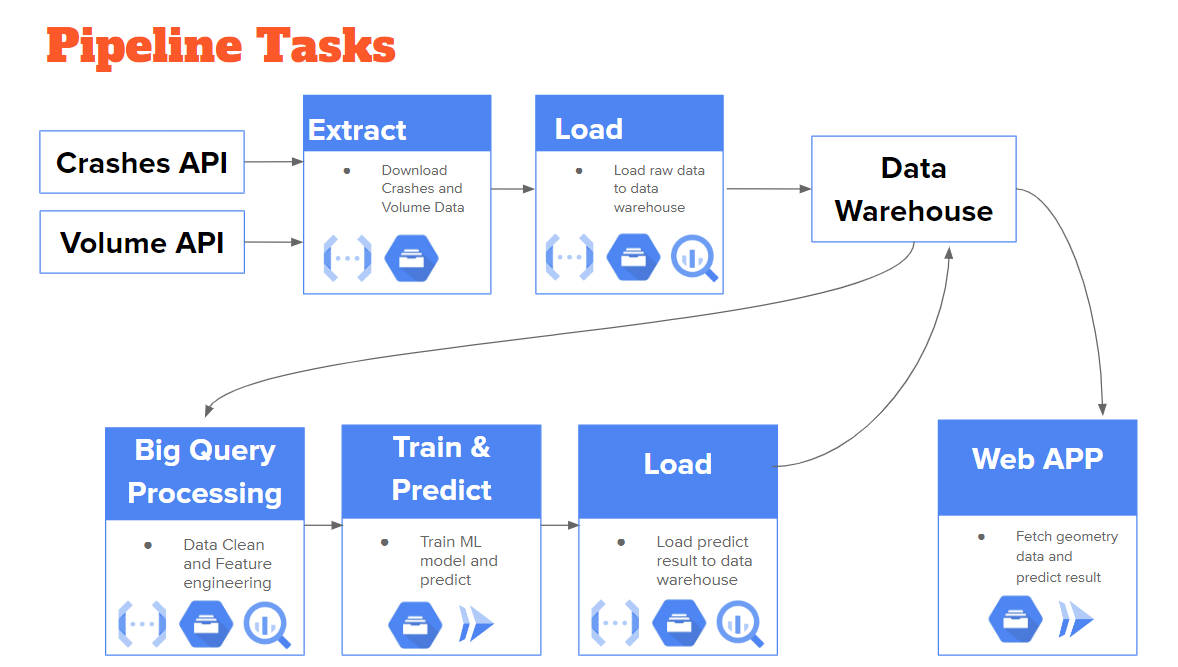
Results
-
Community economic status is strongly associated with child car accident rates. While the p-value of community-level variables in the linear model may not always meet traditional thresholds of statistical significance, the variance in
the multilevel model suggests that a significant portion of the variation in car crash rates can be attributed to communitylevel
factors.
- This research uses MAPE (Mean Absolute Percentage Error) to evaluate the prediction accuracy of multilevel poisson model, which is 0.52. While this indicates that this model is not perfectly accurate, it is still able to effectively identify high-risk areas for car crashes. By comparing the mapping of real car crash history and prediction, we found that the pattern of risk areas identified by multilevel poisson model remained consistent despite the slightly lower predictionaccuracy. This suggests that this model is still able to effectively capture the underlying factors that contribute to car crashes and identify areas where preventative measures should be focused.
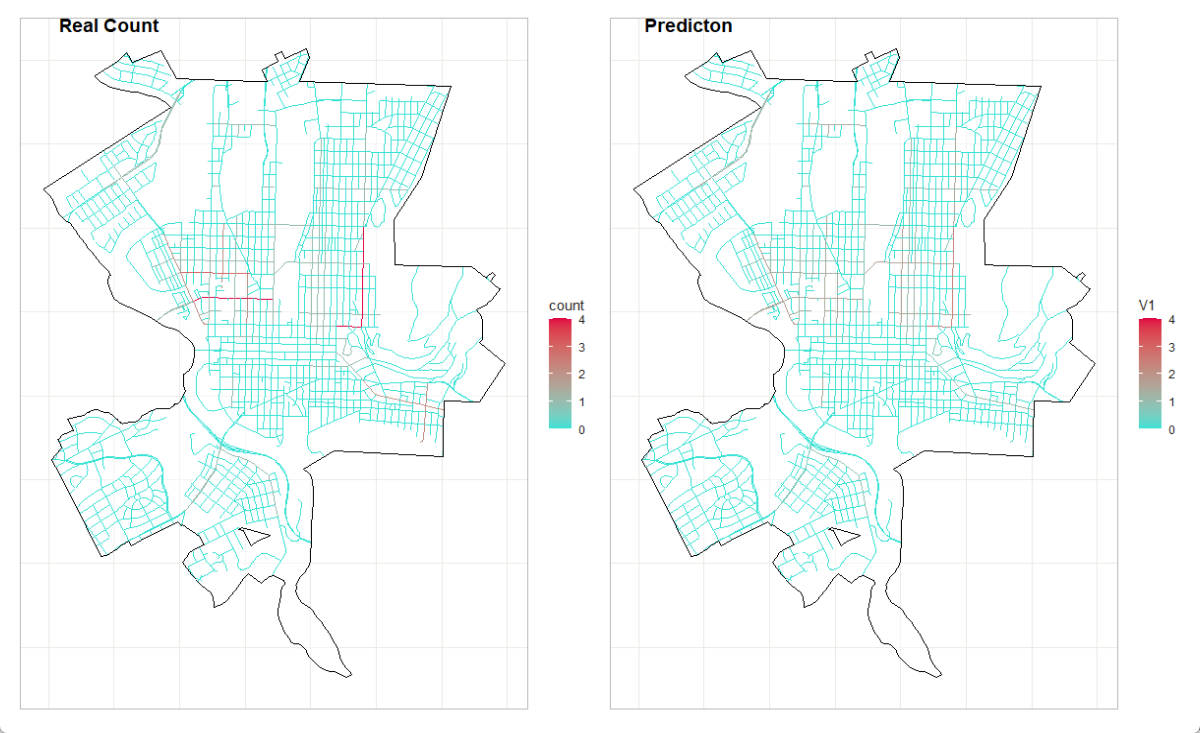
Implication
- Constructed a model for smallsample,
low-probability random
events.
- Informed the development of specific interventions such as increasing the number of stop signs, widening walkways, and designing warning facilities tailored for child pedestrians.
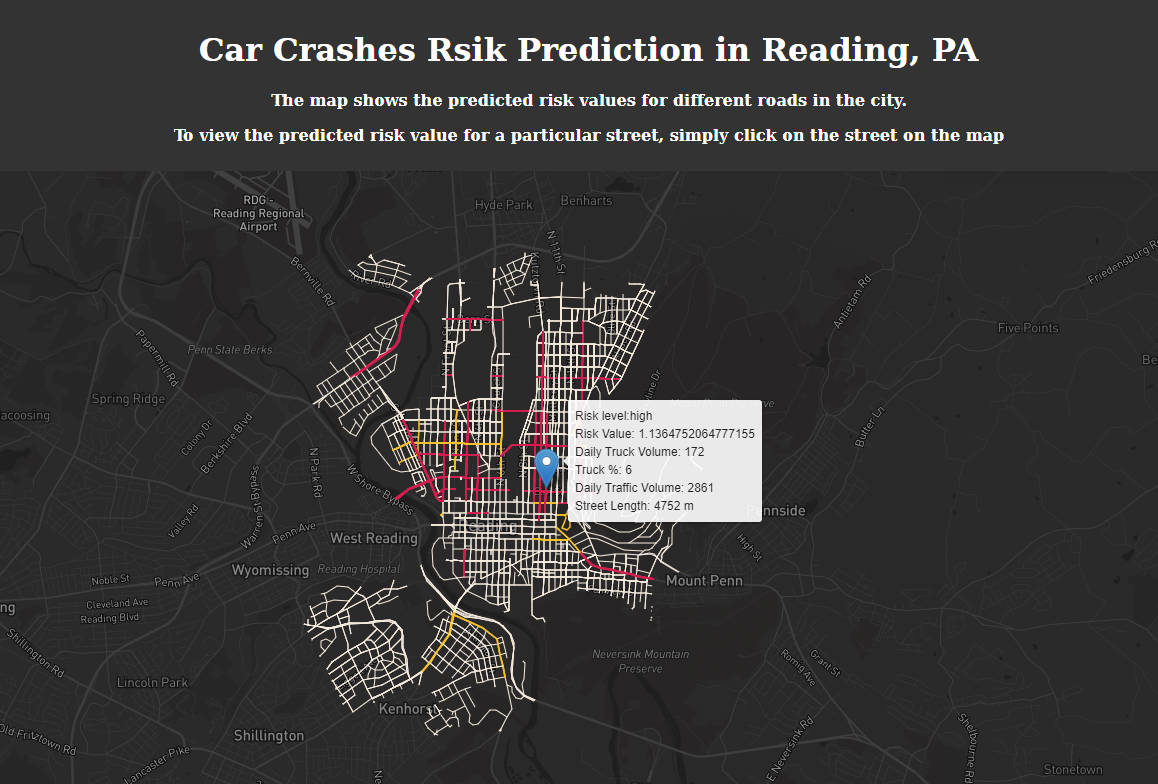